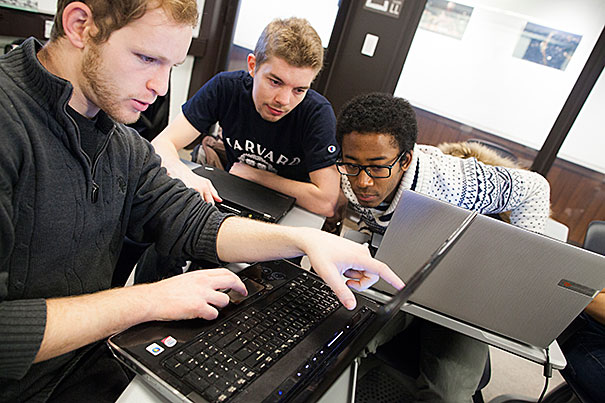
Graduate students Aaron Kaufman (from left), Stephen Merity, and Kevin Kuate Fodouop crunch some numbers in Luke Bornn’s (photo 2) class “Statistics 183, Learning from Big Data.”
Stephanie Mitchell/Harvard Staff Photographer
For big data, big thinking
Statistics class turns to team competition, cooperation to solve complex problems
The information is vast, the challenges daunting, and the tools statistical. But in the end, Harvard’s new class on big data is as much about people as it is about methodology.
The 29 students in “Statistics 183, Learning from Big Data,” work in teams that shift every few weeks, tackling challenges that involve enormous data sets. The students brainstorm and learn from their team members, but they also learn from the work of other teams. Each team posts its solutions to the class projects weekly, and the top three give overviews of their strategies. Students also lecture, presenting a different statistical method to the class each week.
“The learning curve is very steep, but it’s also very exciting,” said Sherrie Wang, a senior biomedical engineering concentrator. “No other class I’ve taken is graded this way and is so project-intensive.”
The course meets twice a week in Quincy House’s newly renovated Stone Hall, under the watchful eye of Assistant Professor of Statistics Luke Bornn and teaching fellow Alex Franks. Bornn said he designed the course to eschew the typical lecture-and-exam format for one that is project-based and emphasizes peer learning. In other words, the class, which is being offered for the first time this semester, is unlike any that Bornn himself has taken.
“This course flips on its head anything that I experienced as a student, intentionally,” Bornn said. “It’s very much all about what they can learn from each other.”
The class is aimed at College seniors who have a background in statistics, and interested graduate students. The peer-learning aspect, Bornn said, makes it important that students come already equipped with an understanding of statistics, as well as some computer programming.
Big data is a hot topic. Statistics, of course, has always been about understanding data, searching for correlations, examining trends, and even understanding what is not known, in the form of uncertainty. Big data, however, is a relatively recent development, a product of modern technology’s ability to gather enormous amounts of information. That ability has brought the promise of a deeper understanding of some of the Earth’s most complex systems, such as the climate system, but also presents problems of handling and extracting meaning from such massive data sets.
Statistics, Bornn said, hasn’t kept pace with technology’s ability to collect information, and even technology runs into limits when data sets are so large they can’t be loaded onto desktop or laptop computers. That leaves open the question of how to handle data sets that contain literally millions of rows.
“Genomics collects petabytes of data. Weather stations are producing data … every minute,” Bornn said. The question is “how do you turn big data problems into small data problems?”
The class seeks to introduce big data to students and give them some tools for handling it. In the past, Bornn said, students getting a typical statistics education would have a good foundation, but would not be ready for the real-world problems they found in their first jobs. The class aims to prepare them for those problems.
“My hope is that they have this ability to say: Here’s a scientific or business problem, here’s a big data set that may or may not be useful, and then be able to go from the raw data to a full write-up,” Bornn said.
The students’ preparation for the real world comes by tackling four online challenges that are open to all comers. The problems and initial data sets are available on Kaggle.com, a data science competition website. They include devising a way to predict the outcome of the NCAA basketball tournament using past data on program strength and tournament seeding; making a wiring map of the brain; classifying Wikipedia articles; and back-casting the initial conditions in an evolved pattern in Conway’s Game of Life, in which a geometric pattern expands — potentially infinitely — according to simple rules.
After learning about the challenge and getting initial data sets, the teams are left alone to brainstorm the most effective approaches. They’re not limited to using just the data they are given, and can search the Web for other information. By mid-semester, the class has done pretty well against outside competition on the Kaggle website, with teams placing in the top 10 among hundreds from around the world in the first two contests.
Andrew Reece, a psychology doctoral student, is taking the course to expand on the statistical background provided by his department’s courses. Reece said he wants to develop an in-depth knowledge of statistical tools so he can apply them to social psychological research. Reece said he appreciates the class’ experimental nature.
“I think he [Bornn] has got this kind of vision that this course is defined by the idea of crowdsourcing, and he’s seeding it into every aspect,” Reece said.
Anthony Liu, a senior mathematics concentrator, said he took the course because he’s interested in the topic, and the peer-learning approach also attracted him. The competition among teams, he said, adds excitement and the challenge of trying to best his friends.
“The big draw of this class was the opportunity to explore these statistical tools in a practical setting and, furthermore, to do so [in] a group … amongst a community,” he said.
Liu said the hardest part of each problem is deciding on an initial strategy among team members. Liu, who has a post-Commencement job lined up at Analytics Operations Engineering Inc., said he expects the work to be familiar because of the big data course.
“It’s basically a professional version of this class,” Liu said.