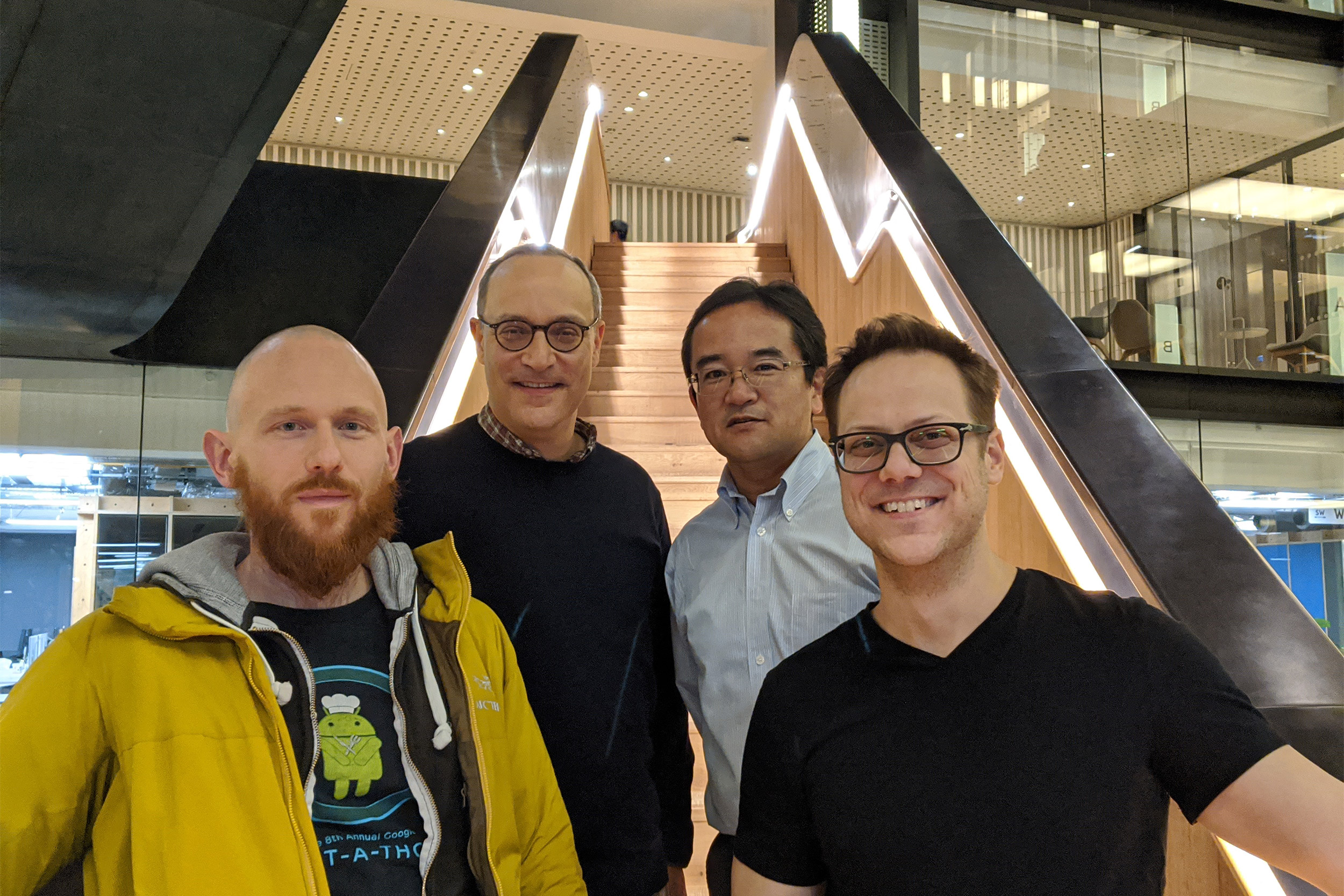
Zeb Kurth-Nelson (from left), Matthew Botvinick, Naoshige Uchida, and Will Dubney at Google in London.
Courtesy of Naoshige Uchida
Beyond Pavlov
Artificial intelligence researchers and neurobiologists share data on how we sort our options and make decisions
Researchers have been able to prove that the brain learns from experience since Russian physiologist Ivan Pavlov conditioned dogs to drool in anticipation of food. In the 100-plus years since, however, scientists studying the brain and the emerging field of artificial intelligence (AI) have been homing in how that happens. A new paper in Nature, “A distributional code for value in dopamine-based reinforcement learning,” goes further in exploring how experience and options are sorted in making decisions.
At issue, explained neurobiologist Naoshige Uchida, professor of cellular and molecular biology and one of the paper’s authors, is “reward prediction” — essentially how well a brain can foresee the risks and rewards of any particular action. Uchida’s lab studies the production of the neurotransmitter dopamine in mice, tracing the chemical decision-making process: Will going after that cheese result in getting stuck in a trap? Is it worth running that maze?
The field of AI has made astonishing progress in recent years but still struggles to reach the complexity of a living brain’s capabilities. Recently, however, said Uchida, AI research has made a breakthrough. Instead of basing AI decisions on learned expectations of “average” outcomes, researchers worked on ways to have their machine brains assess a fuller range of future risk-and-reward scenarios — a probability distribution, which allows for many more factors.
“If you could take into account how risky the outcome is going to be, i.e., knowing the distribution, [that] will help you,” said Uchida. “Your risk preference might change depending on your situation. For instance, if you’re really, really hungry, and you’re going to die if you don’t get a small amount of food now, versus when you’re just mildly hungry.” Such a range of factors, rather than just a static average of a particular behavior’s success, appears to better model how living brains make decisions.
This new approach to understanding learning — including new ways to measure how these decisions are made —“is an exciting development in AI, by itself,” said Uchida. “Then the people at [Google-owned AI company] DeepMind came up with the idea that this could be actually used on the brain.”
The human factor — one of Uchida’s graduate students — provided a crucial link. “My adviser [Uchida] encouraged me to take a summer computational neuroscience course at NYU Shanghai,” recalled neurologist Clara Starkweather, Ph.D. ’18, who met Matthew Botvinick there. Botvinick, director of neuroscience research at London-based DeepMind, had been doing artificial intelligence research on distributional reinforcement learning.
“His group reached out to me to test some of their new theories against data I had collected in mice,” said Starkweather, who is now a graduate student in the Harvard/MIT M.D.-Ph.D. Program at Harvard Medical School. This led to a collaboration of sorts, in which the AI methods were used to help analyze real-life dopamine findings made in Uchida’s lab.
“We actually had the relevant data, and because they had this particular algorithm we could use that to test whether we could decode the distribution. That turned out to be the case,” said Uchida. “This idea of distributional reinforcement learning is consistent with the dopamine data.”
These findings open new pathways for more research in how mice — and humans — learn. They may also have implications for understanding mood disorders, essentially deciphering why certain brains make certain evaluations of risk and reward.
For Starkweather, who describes herself as “an aspiring clinician-scientist,” “having this exposure to collaborating with computational neuroscientists is invaluable.”
“Ideally, experiments can be conducted with the goal of testing a computational hypothesis,” she said. “That allows us to not only report what neurons appear to be doing, but how the brain carries out a computational goal. This way, we can begin to explain disease states where these computations go awry.”
Botvinick, senior author on the paper, noted that both sides benefited and, yes, learned. “In AI, there has been a surge of progress centering on deep learning and reinforcement learning,” he said. “In neuroscience, there has been a revolution in methods, which is giving us access to new realms of data. This study takes advantage of the advances on both sides, using cutting-edge neuroscience techniques to test ideas coming out of the latest AI research. We couldn’t be more excited about the results.”