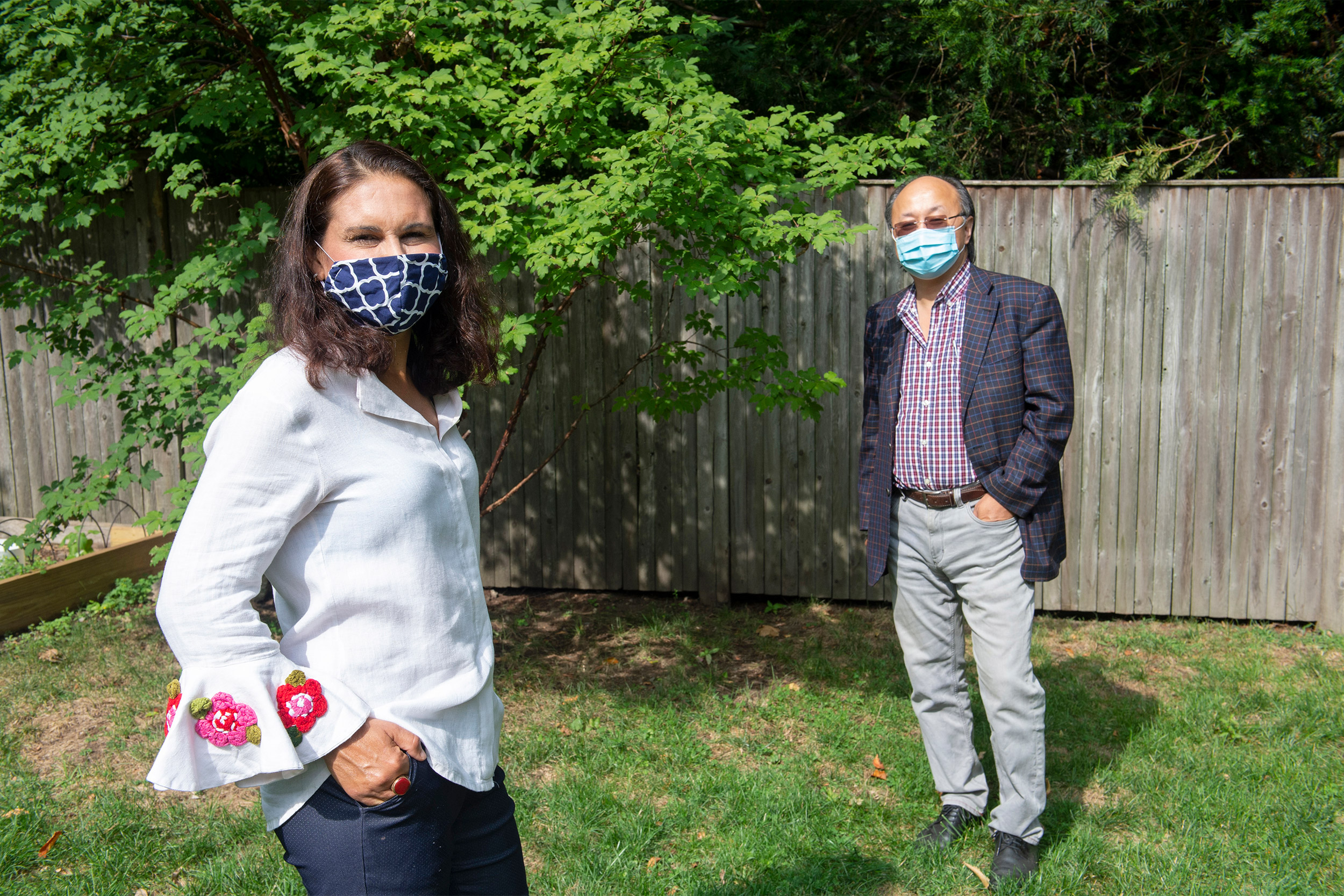
Francesca Dominici, co-director of the Harvard Data Science Initiative, with Xiao-Li Meng, editor in chief of Harvard Data Science Review.
Photos by Jon Chase/Harvard Staff Photographer
An emergency response team for data?
Harvard Data Science Review airs COVID-19 findings
Data science has made key contributions in the battle against COVID-19, from tracking cases and deaths to understanding how populations move during travel restrictions to vaccine design. The Harvard Data Science Initiative is working to support faculty members, students, and fellows in designing and applying the tools of statistics and computer science and creating a community to foster the flow of ideas. The year-old Harvard Data Science Review published a special issue online this summer dedicated to COVID-19 that will be updated with the latest findings, with a goal of fostering innovation and keeping the conversation going about how data science can help meet the COVID-19 challenge. The Gazette spoke with Francesca Dominici, Clarence James Gamble Professor of Biostatistics, Population and Data Science at the Harvard T.H. Chan School of Public Health and co-director of the initiative, and Xiao-Li Meng, the review’s editor in chief and the Whipple V.N. Jones Professor of Statistics in the Faculty of Arts and Sciences, about how data science can be used to meet today’s challenges, and in turn, challenges facing the field.
Q&A
Francesca Dominici and Xiao-Li Meng
GAZETTE: How is data science important to our understanding of and response to COVID-19?
DOMINICI: Data science is on the front page of The New York Times probably every single day. I think that the pandemic has definitely increased the appreciation of data science as an important discipline that can help us solve enormous challenges impacting society. Data science is becoming paramount to understanding almost all of the critical aspects of COVID-19. That includes the development and testing of a COVID-19 vaccine, understanding the factors that slow the rate of infection, understanding the role of airborne transmission — which is critical to understanding whether we can reopen the schools — identifying environmental and socioeconomic factors, and tracking mobility to better understand key behavioral interventions to contain the spread of the virus. Some of my research, for example, is on pollution and COVID-19, which the wildfires in California are making even worse. It’s hard to think about an example regarding COVID-19 that doesn’t have data science methodology and challenges at the forefront, and Harvard faculty across all of the Schools have been doing cutting-edge research at the intersection of data science and COVID-19.
GAZETTE: How has data science helped decision-makers and others think more clearly about uncertainty?
MENG: If there’s any silver lining to COVID-19, it’s that it is making everybody aware of the importance of understanding uncertainty. How do you assess uncertainty? How do you plan under uncertainty? For this special issue on COVID-19, we launched new feature called “Conversations with Leaders,” and the first interview is with President Larry Bacow. We asked him how he used data to plan for Harvard’s shutdown in March and to decide how to reopen this fall. He says it was easier to shut down because the risk was asymmetric: If we closed too early and it turned out to be nothing, he would have gotten laughed at, but leaders get laughed at all the time. But if Harvard closed too late and people died, that is something we can’t live with. Deciding how to open up was much harder because there were a lot more unknowns. Students tend to be younger and impacted less by COVID-19. But President Bacow had to worry about the entire University community — faculty, staff, and different age groups. It was enormously complicated. We did a second conversation with the MIT president, L. Rafael Reif, and we asked him the same question. MIT has designed its dorms to help students interact with each other, which now became a challenge. We talked about how you can talk to experts to understand risk, but due to uncertainty no single person knows for sure. Collectively, we hope we can get a better picture — I don’t think we would ever get a perfect picture — and the Harvard Data Science Review is a place to hear all these different voices, and different perspectives.
“It’s hard to think about an example regarding COVID-19 that doesn’t have data science methodology and challenges at the forefront.”
Francesca Dominici
GAZETTE: A lot of people have been wrestling with uncertainty, but the public may not quite understand the central role that uncertainty has played in this pandemic. Leaders are forced to make decisions based on imperfect, perhaps conflicting, information. Can you talk about how data science helps in situations where there isn’t a yes-or-no answer?
DOMINICI: We all feel the importance of quantifying and communicating uncertainty and embracing the need to make decisions under uncertainty. Unfortunately, some leaders want to dismiss uncertainty in making decisions while data scientists want to acknowledge uncertainty, which doesn’t mean that they [the data scientists] cannot provide new information and guide decision-making. The result is an enormous amount of tension.
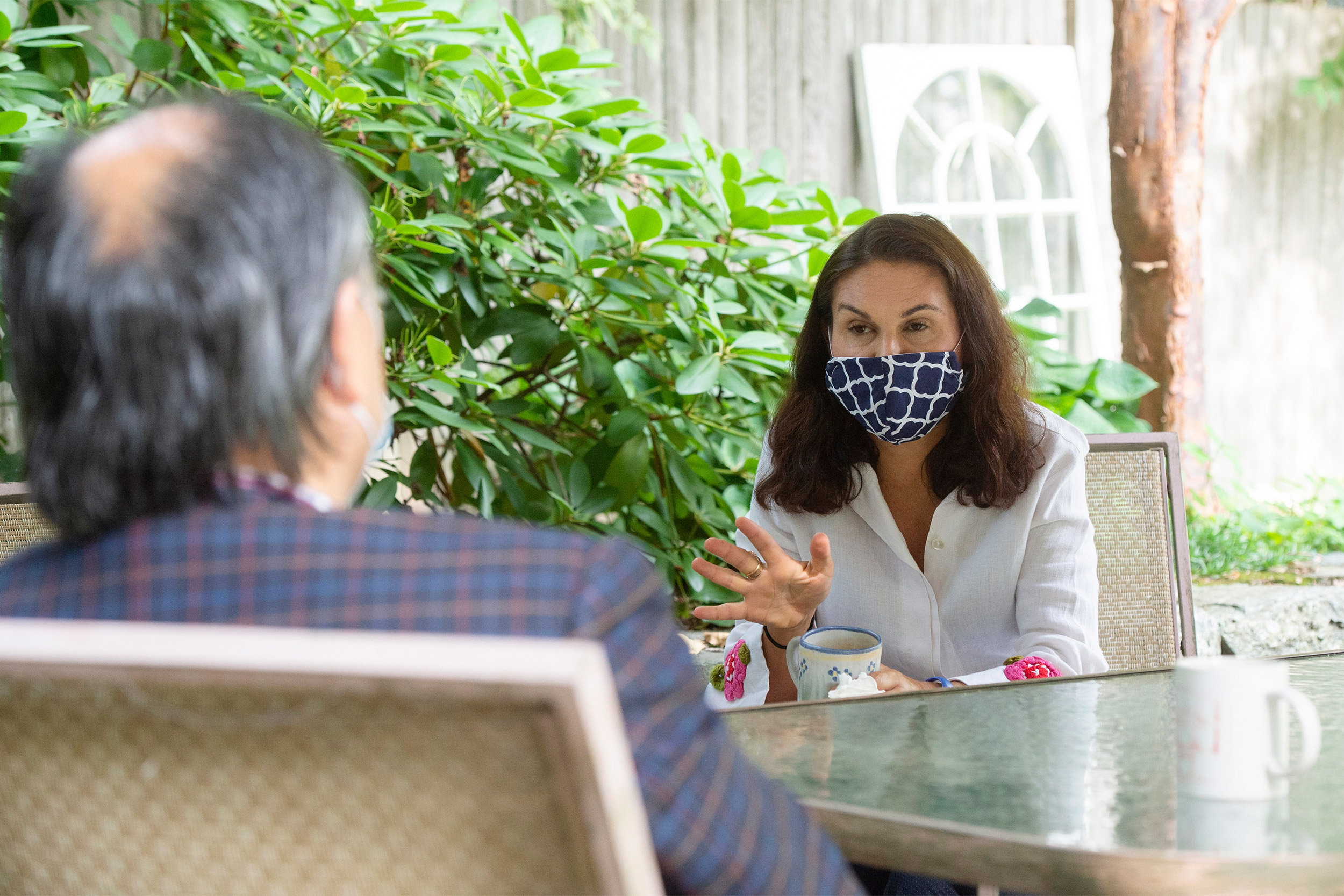
GAZETTE: Is there a misunderstanding that uncertainty means you should dismiss findings — because we’re not sure — even though in your field, uncertainty just means you use all the tools at your disposal to find a likely path, perhaps the most likely path, to success?
MENG: We had this conversation with the head of statistics for BBC News on exactly that point. As data scientists or statisticians, we like to present things called “confidence intervals.” We’re saying, “We’re not sure what it is, but there’s a range.” But ironically, presenting confidence intervals may result in the public losing confidence in us. Many people want one number even though the reality is we can’t produce one number, because even the best possible number comes with so much uncertainty. We had a conversation with the editor in chief of Brief19, Jeremy Faust, a Harvard faculty member and ER doctor. He said it’s incredibly hard to estimate how many people really died from COVID19. You might think that’s a trivial question, but we know for sure very early on in the pandemic that people died whose deaths were not attributed to COVID-19. Now, however, it’s possible that there’s over-attribution, because whenever people die of multiple possible causes, if one of them is COVID-19, then likely that will be reported.
GAZETTE: I know you’re using that as an example of a broader point, but there’s a rousing debate on COVID-19 death estimates. Do you have a sense as to which direction data science is pushing the numbers, higher or lower than official estimates?
MENG: Well, to answer this as a true statistician, I don’t trust any single numbers, because they should be given as a range. Another thing that makes this incredibly hard is data quality. One HDSR paper that has been quoted by the World Health Organization, “On Identifying and Mitigating Bias in the Estimation of the COVID-19 Case Fatality Rate,” deals with multiple sources of statistical bias in calculating case fatality numbers. So, instead of using any single number, let’s play out all these different scenarios and then see what the range of numbers is. In a way, you can already see it in how the media has been constantly revising numbers. Although they’re reporting one number at a time, the revisions are effectively reflecting various kinds of assumed states.
DOMINICI: There are two enormous complications. First of all, this is still evolving because we are still in the middle of the epidemic. These data keep coming so all of these analyses will have to be implemented in a way that can be repeated routinely. But I think the biggest challenge is that when you’re thinking about range, which number you pick within the range has enormous political and economic consequences. This is why the role of data science and the role of the Data Science Review is to be transparent about these challenges, so that when we look retrospectively at data science’s contribution to this topic, it’s clear that we have always been rigorous and we haven’t been partisan in one way or another.
“If there’s any silver lining to COVID-19, it’s that it is making everybody aware of the importance of understanding uncertainty.”
Xiao-Li Meng
GAZETTE: Have there been key data science findings that have not gotten enough attention over the last few months?
MENG: The key findings, which most people in the field would suspect from the very beginning but which have not been emphasized enough, is that the quality of the data is really very low. We all understand no one’s to blame because we’re all struggling and it’s just hard to collect data well when everyone is trying to save lives. Whatever data you can collect, you collect. In the medical community, there are practices for dealing with emergency situations, we have emergency protocols, emergency rooms, etc. But in the data science community, we don’t have this idea of a rapid response team. So when something like this happens we are unprepared. We want to share data without invading privacy, but how do you collect accurate, timely data when people are frantically trying to save lives? For most doctors, they’re not thinking about collecting data, but if you think of the big picture, collecting reliable data is also about saving lives.
Another question I think people are starting to pay more attention to is how do you deal with societal dilemmas like protecting privacy? Tracing people’s movements is definitely helping understand how the pandemic is evolving, but there are enormous privacy issues there. How do you strike the right balance? It used to be that we had guidelines we could work with, but this pandemic is global in scale and different countries have different ways of doing it. One particular article, “Tackling COVID-19 through Responsible AI Innovation: Five Steps in the Right Direction,” is getting lots of attention. This is the longest paper we’ve ever published, over 16,000 words. The author laid down guiding principles for dealing with these complicated issues, difficult problems that really, truly have no unique solution. These are really charged questions and, in the end, these are not problems that data scientists — or any single group — can solve. This is a question for the society: How much trade-off do we want to have?
DOMINICI: To go back to Xiao-Li’s initial point and one which has not been given enough attention, there is no good data science without good data. I think that we are learning but we have to do better in terms of making sure that the data is available. A national registry about individual COVID-19 cases should be made available. Some states are releasing data and some are not. The great majority of research on COVID-19 has been done with data from the Johns Hopkins site. They have been in the forefront, but that data is at the county level for the United States and we’d like to see individual data. That goes back to what Xiao-Li pointed out in terms of mounting an emergency response to gather quality data. There is no easy solution but I think that is something we should work on. We also need an international registry on individual cases and deaths from COVID-19. There are privacy issues about mobility data, but there are fewer issues with respect to case data because they can be de-identified. We need age, race, and gender. Politicians make decisions based on the evidence, so we need to get the best possible evidence out there.
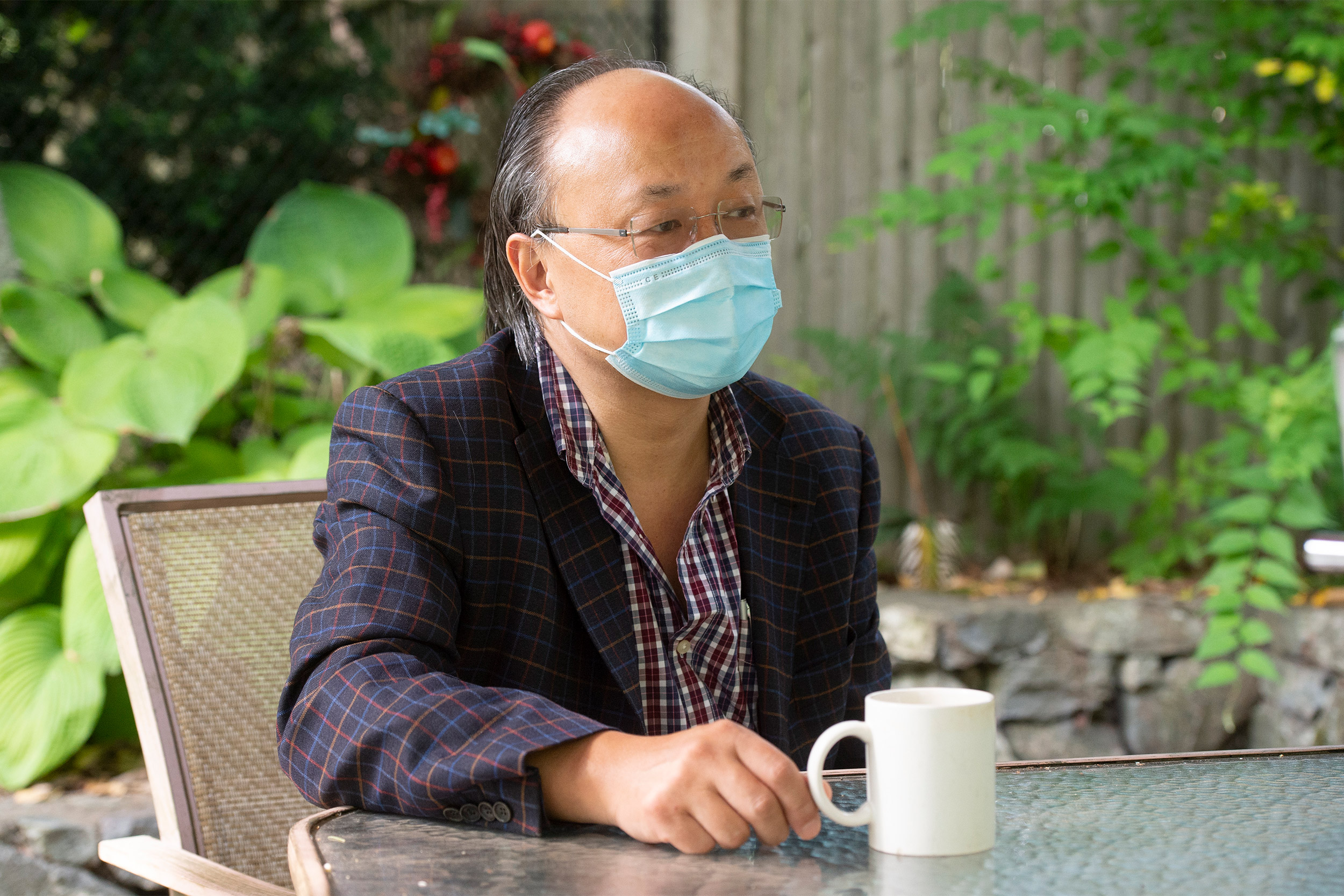
GAZETTE: I was speaking with some folks about artificial intelligence and COVID yesterday and they said the same thing. AI has been more or less a disappointment in our COVID response, and the reason has been because the data quality is very low.
DOMINICI: These algorithms are not intelligent if you don’t train them with high-quality data. You’re going to get artificial stupidity instead of artificial intelligence.
MENG: The problem is that the incentive structures are not right. Collecting data well doesn’t make you a hero, but the data itself is fundamental. Not too long ago, I had a conversation with a few people who were deeply involved in producing national data and statistics. I asked them what big reform they wanted to see, and their first answer was health record data. Collecting this data is not easy because there are other things involved besides the data itself. Lots of us, unfortunately, have multiple diseases, and doctors should determine which is the primary one according to their medical judgment. In most cases that [probably does happen]. But there are incentives to designate as the primary condition one most likely to get the most insurance reimbursement. It’s enormously complicated but most times we don’t hear about the complication, we only hear results: how many cases have been reported. But analyses and predictions are being done without knowing what the underlying numbers really mean. We need a national protocol for doing these things. Another big problem is that you need a workforce that’s trained well enough to be at the forefront of collecting data. They should be able to look at the data, know when “This doesn’t look right,” and understand that the decisions they make in collecting it will directly impact analysis later. Efforts are being made to provide such training as reported in “Change Through Data: A Data Analytics Training Program for Government Employees.”
GAZETTE: Why don’t we talk about the origin of the Harvard Data Science Review? Francesca, why did the initiative decide that having a publication like this was a good idea?
DOMINICI: The Harvard Data Science Review has been a perfect way to communicate data science around the world. To step back for a moment, the Data Science Initiative was launched in 2017, and its goal is to work across Schools and departments to engage and activate data science pioneers in order to address major challenges facing humanities. We wanted to create a highly collaborative network of researchers to multiply the impact of data science discovery in academia and our society. The Data Science Initiative focuses mostly on research and organizes educational conferences. We have a very successful corporate membership program. We wanted to unite our leading computer scientists, statisticians, and domain experts from law, business, public policy, education, medicine, and public health. So we were absolutely delighted when Xiao-Li had this idea of launching a journal. It has become pretty clear that data science is not only statistics; it’s not only computer science; it’s really a new discipline where we need to integrate and leverage expertise across different areas.
GAZETTE: Who is the review’s intended audience? Scientists? The general public?
MENG: Data science has become this enormous ecosystem, as I wrote in my first editorial. In most people’s minds, data science is machine learning, computer science, and statistics. But it includes ethical issues in data collection and analysis, the work of epidemiologists on COVID-19, AI, and topics all the way to quantum computing. Because people working with data science are making advances in their specialty fields, there isn’t a single place to get together to exchange ideas and findings involving data science. As for the Review’s content, we definitely want scholarly research because it’s important that data science is grounded in rigorous theory and methods. We also definitely want to highlight impact, because data science would not exist if it wasn’t for its impact. And, we’re a university, so including data science education is absolutely crucial. When a marketing team asked, “Who is your target audience?” and I answered, “We target everyone,” they said I was crazy. But that’s literally what data science should be.
GAZETTE: Can you walk us through a typical issue?
MENG: The review has four main sections. “Panorama” features pieces from thought leaders on anything data-science-related — philosophy, industry, government. “Cornucopia” features impact, innovation, and knowledge transfer, highlighting how data science can be used in any field. Then “Stepping Stones” has learning, teaching, and communication. Last is “Milestones and Millstones,” where the deeper material runs. We also have columns with different themes. A current one is written by a comedian in the U.K., and she talks about how statistics should “Stop Flaunting Those Curves.” There are columns for pre-college students, for the general public, such as “Can Machine Learning Predict the Price of Art at Auction?” and “Recipes for Success: Data Science in the Home Kitchen.” We have columns on the history of AI, the history of baseball. The goal here is that anybody can pick up this issue, any issue, and find at least one article where they say, “Well, this is interesting.” You can read articles that have no formulas in them, then go to another and think, “Holy cow, how could anybody read this?” Essentially, it’s like a magazine published in multiple languages. What you get out of it depends on who you are.
GAZETTE: Where is the initiative heading over the next year?
DOMINICI: We changed the focus for this year because of what’s happening with COVID-19 and what’s happening with racial discrimination. Those are things that we want to pay attention to. I was impressed because we were contacted by our postdoctoral Harvard data science fellows who said, “We really want to think about the role of data science in addressing racial bias.” So, the goal for the initiative is to pay even more attention to these broader concepts through the lens of data science. We have announced a series of activities looking at responsible data science and data science that reveals discrimination bias. We are devoting a seminar series as well as research funding to using data science to uncover biases and to understand and address the use of badly conceived data science that reinforces bias and inequity. There are many examples where if you’re training machine-learning models used in artificial intelligence on, for example, genetic or diagnostic data from the white population, then you cannot make conclusions regarding what is happening to the Black population. We all know of examples in criminal justice that have exacerbated bias. We also have a very strong corporate members program and another flagship initiative on trust in science: How do we increase public trust in science by leveraging data science? For example, to what degree will people be willing to take the new COVID vaccine?
This interview was edited for clarity and length.